

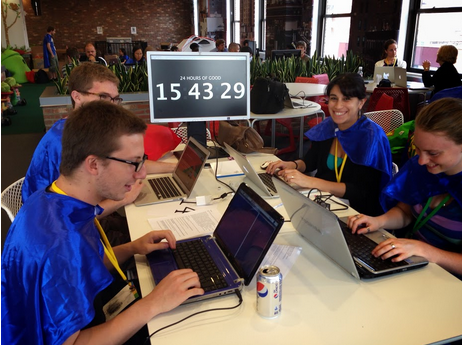
"FAITH: Few-Shot Graph Classification with Hierarchical Task Graphs", International Joint Conference on Artificial Intelligence (IJCAI), 2022 Song Wang, Yushun Dong, Xiao Huang, Chen Chen, Jundong Li."On Structural Explanation of Bias in Graph Neural Networks", ACM SIGKDD Conference on Knowledge Discovery and Data Mining (KDD), 2022 Yushun Dong, Song Wang, Yu Wang, Tyler Derr, Jundong Li."Learning Causal Effects on Hypergraphs", ACM SIGKDD Conference on Knowledge Discovery and Data Mining (KDD), 2022 Jing Ma, Mengting Wan, Longqi Yang, Jundong Li, Brent Hecht, Jaime Teevan."Task-Adaptive Few-shot Node Classification", ACM SIGKDD Conference on Knowledge Discovery and Data Mining (KDD), 2022 Song Wang, Kaize Ding, Chuxu Zhang, Chen Chen, Jundong Li.His research interests are generally in data mining and machine learning, with a particular focus on graph mining, causal inference, and algorithmic fairness. As a result of his research work, he has published over 100 papers in high-impact venues (including KDD, His group's research is generously supported by NSF (CAREER, III, SaTC, SAI), JLab, JP Morgan, and Cisco.
#JING DONG GOOGLE SCHOLAR SOFTWARE#
degree in Software Engineering at Zhejiang University in 2012. degree in Computer Science at University of Alberta in 2014, and B.Eng. degree in Computer Science at Arizona State University in 2019, M.Sc. Jundong Li is an Assistant Professor in the Department of Electrical and Computer Engineering, with a joint appointment in the Department of Computer Science, and School of Data Science. Engineering Technologies for a Sustainable and Connected Worldīio Ph.D.Many new and interesting concepts are presented in this text that we hope the readers will find useful and inspiring. This book is especially written for graduate students and research engineers who work on noise reduction for speech and audio applications and want to understand the subtle mechanisms behind each approach. The other objective of Noise Reduction in Speech Processing is to derive all these well-known techniques in a rigorous way and prove many fundamental and intuitive results often taken for granted. The focus is on the most useful approaches, i.e., filtering techniques (in different domains) and spectral enhancement methods. One of the objectives of this book is to present in a common framework an overview of the state of the art of noise reduction algorithms in the single-channel (one microphone) case. This cleaning process is often called noise reduction and this topic has attracted a considerable amount of research and engineering attention for several decades. As a result, the microphone signal has to be cleaned up with digital signal processing tools before it is stored, analyzed, transmitted, or played out. Noise is everywhere and in most applications that are related to audio and speech, such as human-machine interfaces, hands-free communications, voice over IP (VoIP), hearing aids, teleconferencing/telepresence/telecollaboration systems, and so many others, the signal of interest (usually speech) that is picked up by a microphone is generally contaminated by noise.
